Consulting and Training for fleXality – Climate tech Startup based on Machine Learning
DigitalMara conducted training sessions for the client’s internal tech team. fleXality developed a proprietary algorithm based on Machine Learning and needed additional knowledge on how to assemble all components into a unified system and deploy it into the cloud.
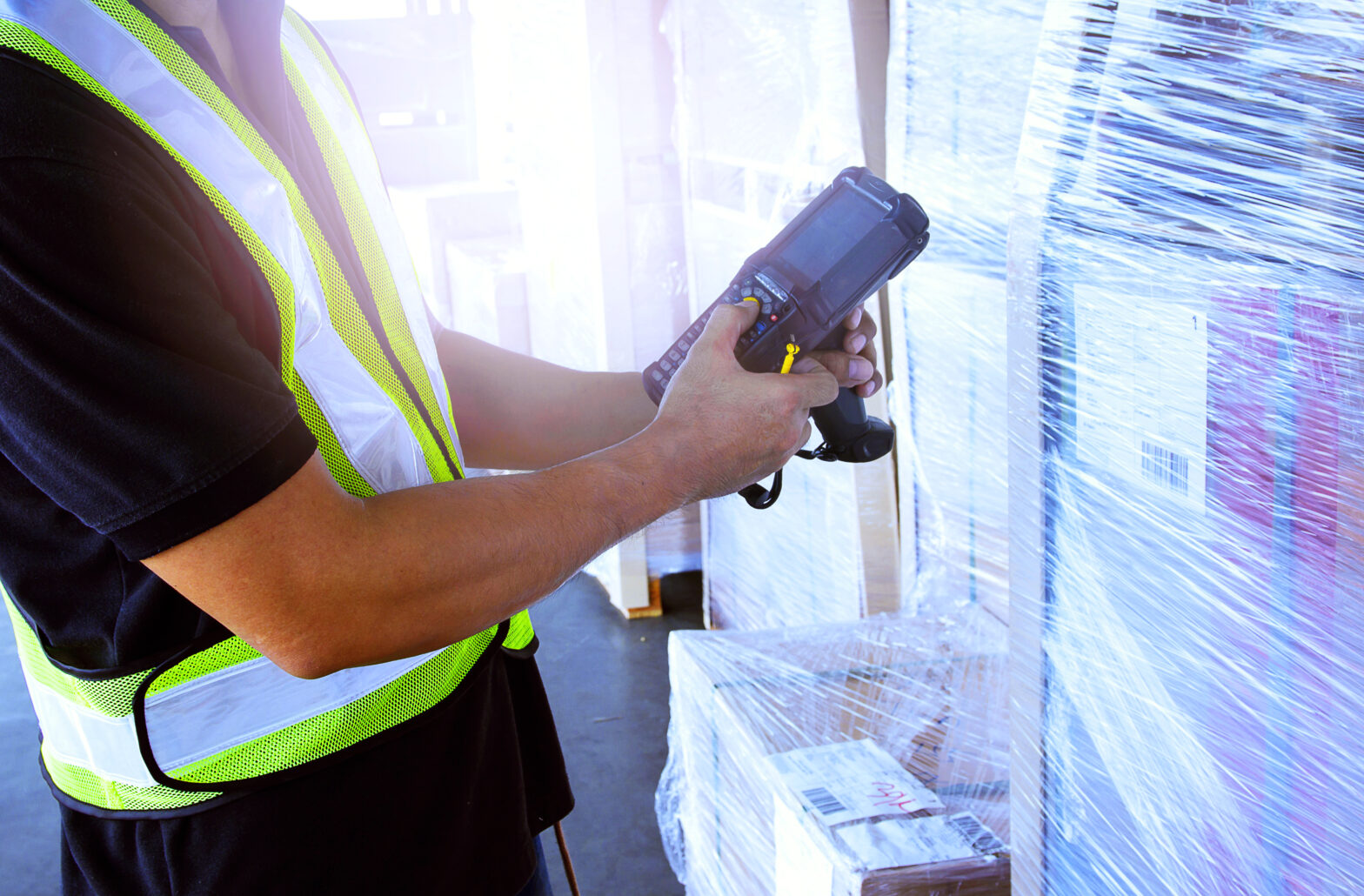
About the client
fleXality, a German climate tech startup, offers data-driven solutions to optimize energy expenditures for cold store plants. The goal is to reduce power costs together with CO2 emissions by shifting the consumption of energy for cold production into phases of lower prices and higher ratios of renewable energies in the power market for this purpose, they developed their own algorithm based on Machine Learning (ML). The algorithm formed the basis of the system that collects and analyzes data, predicts energy demands for cold production and gives recommendations on cost optimization based on physical, operational, and processual parameters.
Approach
The client wanted to review internal processes and get consultancy from specialists who know the specifics of such projects. fleXality wanted to extend their knowledge on how to compile an entire system from components and deploy it in production.
We assessed a technical part within the tasks that needed to be solved and created a training plan. Our Senior MLOps engineer conducted several training sessions as video conferences during the month. According to the plan for each session the list of questions and answers was prepared. There were also questions on the go, and our engineer answered them all.
During the sessions, we discussed building ML models and pipelines in the cloud and local network, created examples of pipelines for models, improvements for the general architecture of pipelines in production and conducted deep dives into the nuances of MLflow.
Results
As a result of training, the client improved their building of pipelines, their ML architecture, and the interaction of components with each other. Now they can deploy the system into production via state-of-the-art processes.